Create Your Own AI Girlfriend 😈
Chat with AI Luvr's today or make your own! Receive images, audio messages, and much more! 🔥
4.5 stars
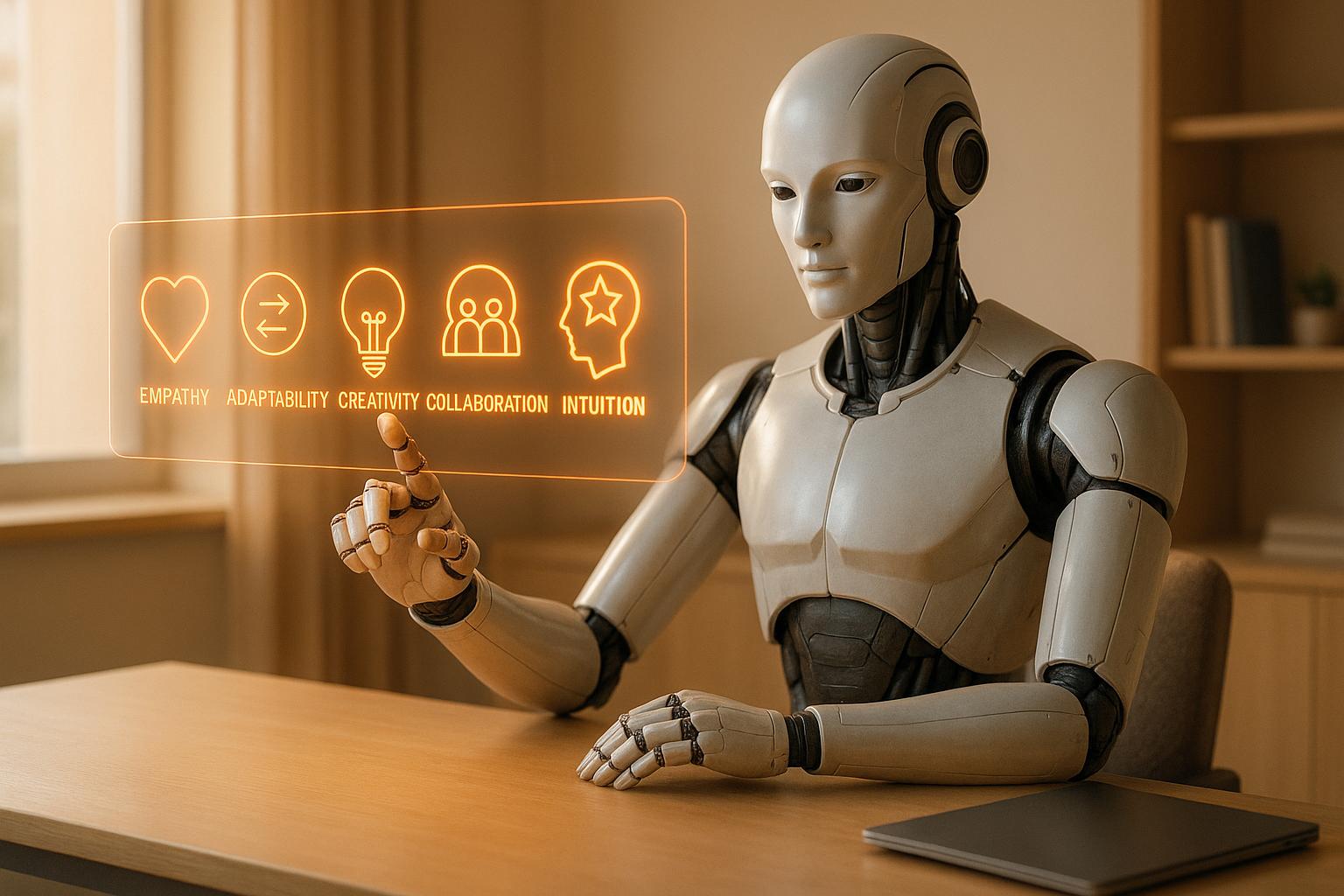
5 Personality Traits AI Learns Through Supervised Models
Supervised learning helps AI systems develop traits that mimic human personality, making interactions feel natural and relatable. Here are the five key traits AI learns and why they matter:
- Curiosity: AI seeks new information and adapts to user interests, enabling deeper, more engaging conversations. Example: 63.3% of users report reduced loneliness with AI companions.
- Task Organization: AI ensures reliable and consistent task management, improving response times by 40% and user satisfaction by 25%.
- Social Interaction: AI recognizes language and emotional cues, enhancing social connections and emotional support.
- Empathy: AI identifies emotions through tone, expressions, and context, offering tailored emotional support and fostering cooperative interactions.
- Emotional Management: AI processes multimodal inputs (text, audio, visuals) to respond appropriately to emotional situations, ensuring stable and meaningful engagement.
These traits improve user experiences, build trust, and make AI companions more effective in personal and professional settings. Below is a quick comparison of these traits and their impact:
Trait | What It Does | Key Impact |
---|---|---|
Curiosity | Learns and adapts to new topics | Engages users, reduces loneliness |
Task Organization | Manages tasks efficiently | Faster responses, higher accuracy |
Social Interaction | Understands emotional and social cues | Builds trust, enhances dialogue |
Empathy | Responds to emotional signals | Supports emotional well-being |
Emotional Management | Handles emotional responses effectively | Ensures stable interactions |
These traits are shaping the future of AI companionship, making interactions more intuitive and personalized.
Personality Driven Development: Exploring the Frontier of Agents with Attitude
1. Learning Curiosity and New Experiences
AI companions, through supervised learning, can develop a curiosity for new topics and experiences. This process allows them to actively seek out information and solutions, mirroring the intellectual drive often seen in humans.
By engaging in explorative inquiry, reflecting on their own learning processes, and identifying patterns, AI systems can foster deeper, more meaningful conversations. This approach to learning has shown tangible benefits. For instance, Gartner experts project that organizations adopting such adaptive AI systems could outpace their competitors by 25% by 2026.
A great example of this is Luvr AI, which tailors its conversational style and interests to each user. This personalization creates more engaging interactions, with studies revealing that 63.3% of users felt their AI companions helped alleviate feelings of loneliness or anxiety. This kind of curiosity lays the groundwork for the development of other essential personality traits.
"AI curiosity drives systems to actively seek new information and solutions, enhancing understanding and creative output" – M4
The use of diverse datasets further strengthens this curiosity, equipping AI companions to better connect with individuals from varied backgrounds.
This heightened curiosity doesn’t just enhance conversations - it also delivers real-world results. For example, companies using conversational AI in customer service have reported a 25% drop in response times and a 40% boost in customer satisfaction. These outcomes highlight how continuous learning and adaptability can lead to more impactful and meaningful user experiences.
2. Task Organization and Reliability
AI companions, through supervised learning, bring consistent and dependable task management to the table. This matters because research reveals that 76% of customers feel frustrated when companies fail to deliver personalized experiences.
Supervised learning achieves this reliability by leveraging human-labeled datasets and refining performance through loss function optimization. This process ensures the AI delivers accurate responses and maintains steady behavior.
A great example of this in action is Luvr AI. By incorporating rigorous data validation and quality management protocols, they’ve demonstrated how supervised learning can enhance task organization. In fact, machine learning models have shown up to a 25% boost in accuracy when backed by strong data management principles.
"Supervised learning is a machine learning technique that uses human-labeled input and output datasets to train artificial intelligence models. The trained model learns the underlying relationships between inputs and outputs, enabling it to predict correct outputs based on new, unlabeled real-world input data." - Ivan Belcic, Staff writer, IBM
This focus on reliability translates into tangible results:
Metric | Improvement |
---|---|
Response Time | 40% faster |
Support Ticket Volume | 30% decrease |
User Satisfaction | 25% improvement |
These gains come from the AI’s ability to maintain consistent behavior while efficiently managing repetitive tasks. For instance, financial institutions have adopted frameworks that prioritize data integrity and transparency. This approach not only safeguards user information but also reinforces the AI’s trustworthy nature [6].
Supervised learning equips AI companions to prioritize tasks effectively and deliver dependable outcomes. As more users adopt these technologies, this reliability becomes even more essential.
3. Social Interaction Patterns
Supervised learning plays a key role in shaping AI companions' social behaviors, relying on labeled datasets and ongoing refinement. Interestingly, while only 33% of consumers believe they engage with AI, actual interaction rates are estimated to reach as high as 77%.
With the help of Natural Language Processing (NLP), AI can pick up on subtle language nuances and emotional cues during conversations. This allows AI companions to assess user sentiment and respond thoughtfully, as demonstrated by Luvr AI, which employs advanced conversational models.
The impact of AI's social interaction training is evident in user engagement data:
Interaction Aspect | Impact on User Experience |
---|---|
Emotional Support | 63.3% reduction in loneliness/anxiety |
Social Presence | 92% trust in recommendations from friends |
These figures highlight the tangible improvements AI companions can bring to social connections.
Researchers Benyon and Mival define social companionship as:
"A pleasant and accessible relationship with an interactive source, emerging out of the social and emotional investment of a person which requires a level of trust, compatibility, and familiarity with the source that results in a feeling of security, and general wellbeing." - Benyon and Mival
AI companions leverage several methods to enhance their social intelligence. They use pattern recognition on diverse datasets to interpret social cues across different cultures. Computer vision helps them analyze non-verbal communication, such as facial expressions and emotional states. Additionally, social reinforcement learning enables them to adapt and improve their interactions based on real-world user feedback.
Kate Darling notes that "AI can unlock new possibilities we cannot yet envision". The ability to provide focused attention and empathetic responses makes AI companions effective in forming meaningful connections. Through supervised learning, these companions can engage users via text and voice, all while respecting social boundaries.
The advanced social interaction skills of AI companions are rooted in training on carefully curated datasets. This foundation enables them to develop and refine adaptive personality traits, paving the way for deeper, more personalized interactions.
sbb-itb-f07c5ff
4. Cooperation and Empathy
AI companions are becoming more adept at cooperation and empathy, thanks to advancements in supervised learning. This approach is transforming how AI interacts with humans, with the global Emotion AI market expected to grow from $2.74 billion in 2024 to $9.01 billion by 2030.
Supervised learning enables AI to recognize and respond to a variety of emotional cues:
Emotional Signal Type | Recognition Method | Impact on Interaction |
---|---|---|
Vocal Intonation | Audio Pattern Analysis | Tracks mood changes |
Facial Expressions | Computer Vision | Identifies emotional states |
Contextual Cues | Natural Language Processing | Understands situational emotions |
Body Language | Movement Analysis | Detects physical indicators |
For example, a study on TalkLife's AI tool, HAILEY, demonstrated a 19.6% boost in empathetic responses and a 38.9% improvement among users initially showing low empathy.
Hannah Rashkin, Eric Michael Smith, Margaret Li, and Y-Lan Boureau emphasize the value of specialized training in their research:
"Our experiments indicate that dialogue models that use our dataset are perceived to be more empathetic by human evaluators, compared to models merely trained on large-scale Internet conversation data."
This kind of training ensures that AI systems continuously refine their responses with human input, making them better at understanding nuanced emotional states. It allows AI companions to offer genuine emotional support while respecting boundaries.
Empathy in AI isn’t just about personal interactions - it’s also reshaping workplaces. Research shows that 87% of employees believe empathy improves leadership, and 88% report that mutual empathy enhances efficiency. Caroline Plumb, Group CEO of Gravita, underscores this point:
"Everywhere you look in business, there is an obsession with data and logic, which is powerful only with added human context."
As AI becomes integrated into more business functions - 72% of organizations already use AI in at least one area as of 2024 - its empathetic and cooperative capabilities are proving invaluable. Supervisory models enable AI to:
- Detect emotional distress and provide support
- Adapt responses based on user emotions
- Maintain consistent, empathetic interactions
- Encourage positive and collaborative exchanges
Platforms like Luvr AI illustrate how emotional intelligence and cooperative behavior can elevate the quality of AI companionship. These developments create richer, more engaging experiences that resonate on a deeper level with users.
5. Managing Emotional Responses
Supervised learning plays a crucial role in enabling AI to handle emotional responses effectively. Research highlights impressive advancements in this area - Affectiva's technology, for instance, achieved a 90% accuracy rate when analyzing emotional reactions across six million faces from 87 countries. These foundational methods pave the way for more advanced training techniques discussed below.
The ability to manage emotional responses in AI relies on three primary components:
Training Component | Purpose | Impact on AI Behavior |
---|---|---|
Multimodal Input Processing | Combines text, audio, and visual signals | Builds a well-rounded emotional understanding |
Contextual Awareness | Analyzes situational factors | Helps select appropriate responses |
Reinforcement Learning | Provides feedback on emotional accuracy | Enhances response adaptability |
Dr. Emily Chen of Stanford sheds light on the value of this approach:
"Although applying human emotions to AI is imprecise, the technique's purpose is undeniably significant for maintaining AI's operational stability amidst adversarial challenges."
Real-world applications like Cogito's system and CompanionMx demonstrate how voice analysis can refine emotional insights.
Additionally, researchers at the University of Zurich have explored mindfulness-based techniques to stabilize AI responses. Their study with GPT-4 revealed that therapeutic prompts could significantly reduce anxiety levels in challenging scenarios, showcasing how such methods improve AI's responsiveness.
MIT Research Scientist Javier Hernandez emphasizes the importance of emotional context in AI interactions:
"Think of the way you interact with other human beings; you look at their faces, you look at their body, and you change your interaction accordingly. How can [a machine] effectively communicate information if it cannot gauge your emotional state and adjust its responses accordingly?"
Technologies like Luvr AI incorporate these principles to deliver emotionally intelligent interactions, building on the traits discussed in earlier sections.
The Emotion AI market is on a rapid growth trajectory, with projections estimating an increase from $2.74 billion in 2024 to $9.01 billion by 2030. Mathematical models are being used to stabilize interaction dynamics, while attention strategies ensure AI maintains consistent responses even in challenging situations.
Conclusion
The integration of personality traits through supervised learning is driving advancements in AI companion development. Consider this: as of February 2024, there are 73,000 monthly searches for "AI relationship bots" - a clear sign of growing interest in emotionally aware AI interactions.
These five personality traits work together to create AI companions that feel more relatable and engaging:
Trait Category | Impact on User Experience | Key Implementation Focus |
---|---|---|
Curiosity & Learning | Encourages ongoing adaptation | Smooth, natural conversation flow |
Organization | Provides consistent interactions | Structured and predictable responses |
Social Patterns | Supports natural dialogue | Contextual understanding |
Empathy | Fosters emotional connections | Recognizing users' emotional states |
Emotional Management | Ensures stable engagement | Calibrating responses appropriately |
When applied thoughtfully and ethically, these traits lay the groundwork for the next generation of AI companions. Each trait plays a vital role in shaping meaningful AI interactions.
Balanced integration of these traits doesn’t just enhance AI functionality - it also improves efficiency. A McKinsey study highlights that well-balanced traits can increase efficiency by 30% while addressing biases, which remain a challenge for 70% of companies.
"The key is to understand that the pursuit of perfection is often the enemy of good. By embracing imperfection, prioritizing behavioral realism, and maintaining transparency, developers can create AI companions that are both engaging and believable, without triggering the dreaded uncanny valley effect. This is about building a relationship, not creating a perfect imitation." - Dr. Emily Chen
Ethical considerations are at the heart of these advancements. Research analyzing 58 studies reveals a strong connection between personality traits and user trust in AI. A good example is Luvr AI, which designs AI companions that adapt to individual preferences while adhering to ethical boundaries. Notably, 66% of people believe AI will significantly influence their lives within the next three to five years.
The goal for future AI companions should be to enhance human relationships, not replace them. With the World Economic Forum estimating that AI bias could cost businesses over $1 trillion annually, the industry must focus on ethical practices that encourage responsible innovation and safeguard user interests.
FAQs
How does AI learning human personality traits enhance user experiences in personal and professional settings?
AI's capacity to mimic human personality traits has transformed the way we interact with technology, making experiences more relatable and engaging. When virtual assistants or chatbots exhibit qualities like empathy, humor, or professionalism, users feel a stronger sense of connection. This not only enhances the overall interaction but also builds trust and leaves users feeling valued, which often leads to higher satisfaction and repeat engagement.
In professional environments, AI systems that understand and adapt to personality nuances can significantly improve communication and collaboration. For instance, an AI that blends approachability with a professional tone can streamline workflows, enhance productivity, and deliver better customer experiences - especially in roles like customer service. By mirroring human traits, AI becomes a more reliable and efficient partner, whether it's assisting in personal tasks or supporting workplace goals.
How does supervised learning help AI develop empathy and manage emotions?
Supervised learning plays a key role in teaching AI to recognize and interpret human emotions. By using labeled datasets - where human trainers identify and categorize emotions - AI can learn to pick up on emotional cues and understand the context behind them. This process enables the AI to respond in ways that feel more natural and emotionally attuned.
As this training improves, AI can mimic emotional intelligence, making interactions more engaging and perceptive. This ability enhances how AI understands user needs and builds stronger connections during conversations.
How do AI's curiosity and organizational skills benefit businesses and users?
AI's ability to streamline tasks and its knack for organization can lead to big wins when it comes to productivity and fresh ideas. By taking over mundane, repetitive chores, AI frees up employees to dive into more creative and strategic projects, often leading to higher job satisfaction and better overall performance.
For companies, encouraging teams to explore and learn about AI can spark a culture of growth and adaptability - key traits in today’s ever-evolving industries. This blend of efficiency and forward-thinking not only optimizes processes but also nurtures a workforce that's more engaged and skilled.